(a)
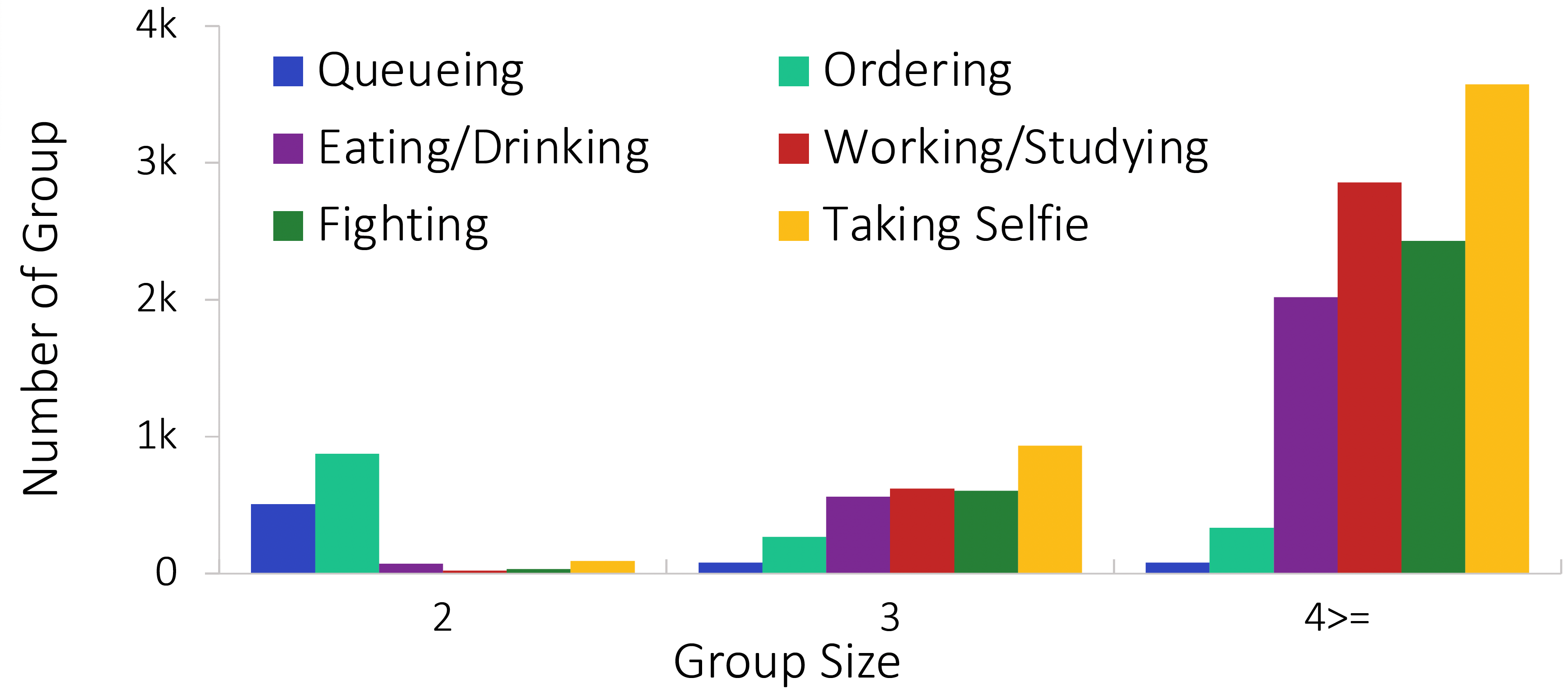
Place A | Place B |
Place C | Place D |
Place E | Place F |
Café dataset is taken at six different cafes to capture realistic daily activities, and each of them exhibits multiple non-singleton groups performing their own activities as well as outliers, presenting more practical evaluation scenarios for GAD.
Group activity detection (GAD) is the task of identifying members of each group and classifying the activity of the group at the same time in a video. While GAD has been studied recently, there is still much room for improvement in both dataset and methodology due to their limited capability to address practical GAD scenarios.
To resolve these issues, we first present a new dataset, dubbed Café. Unlike existing datasets, Café is constructed primarily for GAD and presents more practical evaluation scenarios and metrics, as well as being large-scale and providing rich annotations.
Along with the dataset, we propose a new GAD model that deals with an unknown number of groups and latent group members efficiently and effectively. We evaluated our model on three datasets including Café, where it outperformed previous work in terms of both accuracy and inference speed. Both our dataset and code base will be open to the public to promote future research on GAD.
Examples of videos in Café. The videos were taken at six different places and four cameras with different viewpoints.
Comparison between Café and other datasets for group activity understanding.
(a)
(b)
(c)
(a) Group population versus group size per activity class. (b) Distribution of the number of actors in each video frame. (c) Comparison between Café and the other datasets in terms of group size.
(Left) Overall architecture of our model. (Right) Detailed architecture of the Grouping Transformer.
Quantitative results on Café. The subscripts of Group mAP mean Group IoU thresholds. We mark the best and the second-best performance in bold and underline, respectively.
Qualitative examples of prediction by our model on Café. Boxes with the same color belong to the same group. All outliers are marked with teal color. Each color represents different group activity class (queueing: indigo, ordering: red, eating: orange, working: yellow, fighting: violet, selfie: pink, outlier: teal).
Input frames | Ours | Ground-truth |
![]() |
![]() |
![]() |
![]() |
![]() |
![]() |
![]() |
![]() |
![]() |
![]() |
![]() |
![]() |
![]() |
![]() |
![]() |
![]() |
![]() |
![]() |
@article{kim2023towards,
title={Towards More Practical Group Activity Detection: A New Benchmark and Model},
author={Kim, Dongkeun and Song, Youngkil and Cho, Minsu and Kwak, Suha},
journal={arXiv preprint arXiv:2312.02878},
year={2023},
}